Infometry Data Quality and Enrichment Suite
In many companies, a lack of management awareness and support for data quality continues to be a problem. Once again, no “silver bullet” solution can turn your dirty data into good quality. While there are data cleansing tools that can help, it still takes the knowledge of a business person to define the intended meaning and the intended content of each data element.
These have to be documented as business rules before they can be fed to any data-cleaning tool or business rules engine. Infometry combines proprietary algorithms for data cleaning, harmonization & enrichment as SAAS or on-premise offering. The AI platform, coupled with economic ontology, gives you up to 70% discount in time & fee with an excessive diploma of accuracy, 90% and above.
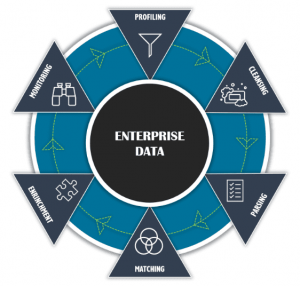

Our Data Quality and Enrichment Solutions
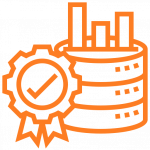
Data Quality and Cleansing
Automates data cleansing – guidelines for data quality standards for diverse tables and columns. An analyst can replace standards and parameters as required, and the system learns from the Analyst’s actions.
Data Quality Dashboard
Visualize standard DQ, table/column unique DQ outcomes. Identify, extract & curate anomalous statistics with the use of DQ dashboards.
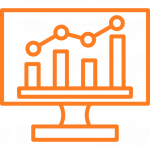
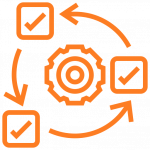
Various Pre-processing Options
Standardize deal with components (e.g.rd -> road, av, ave ->avenue etc.) and commercial enterprise suffixes for the company. Combine and derive new attributes.
Digitization and Reconciliation of Documents
Support for pdf, image, excel, jpeg, different formats and Wide coverage of files, namely, Accounting statements, and different kinds with the ability to educate on new files quickly. Auto-correction of mistakes and advice with pre-built reconciliation modules.
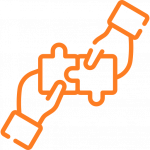
Why Data Quality & Enrichment Solutions?
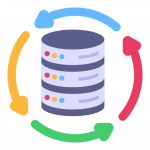
Help enhance data quality & enrichment for enterprises
Minimize duplicates, fill in gaps and refresh stale, outdated information with comprehensive data coverage and quality.
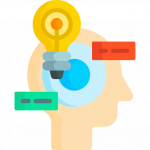
Access insights quickly and with less effort
Receive multiple reports in one efficient file. Bulk files can be delivered as quickly as in just a few hours.
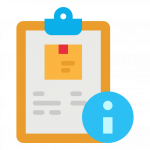
Protect your data with secure file delivery
We offer peace of mind by protecting your customer data. Your files are securely delivered through an encrypted self-service portal or SFTP.
Why Choose us?
Updating and optimizing your data using tools and modules is essential to any Data Quality and Enrichment process. To augment your data enrichment efforts, it is imperative to have a platform that takes advantage of other internal and external sources to achieve continuous data updates.

- We allow users to continuously monitor data quality with auto-thresholds, benchmarking, and actionable alerts to manage the need for data enrichment.
- We improve your team's understanding and process for data enrichment based on data classification rules and principles.
- Get predictive analytics modelling services to define the parameters of your data enrichment project.
- We help you implement a continuous and consistent data enrichment process with ML-assisted data Search and Querying.
- Integrate our data enrichment API solution at once into your website and different applications.
- Get real-time insight into consumer wishes and preferences.
Let's Craft Your AI and Data Quality & Enrichment Journey Together!
Frequently Asked Questions
Data enrichment adds information to existing data sets to make the data more valuable and useful. This additional information can come from various sources, such as external databases, third-party APIs, and manual input.
Examples of data enrichment include:
Geocoding: Adding latitude and longitude coordinates to address data, allowing for location-based analysis and mapping.
Enriching data with external data sources: Joining a dataset with an external data source, such as social media data, weather data, or demographic data, allowing for a complete picture of the data.
Data normalization: Transforming data into a standard format, such as standardizing date formats or converting measurement units.
Data validation: Verifying that data is accurate and complete.
Annotation: Adding notes or comments to data to provide context or explain its meaning.
The ultimate goal of data enrichment is to make data more actionable, informative and accurate by adding context and additional insights, which can be used for better decision-making, analysis and reporting. Data enrichment can be done using various tools and techniques, such as data integration and pipeline tools, data quality tools, and machine learning algorithms.
Data quality refers to accuracy, completeness, and consistency. It encompasses a set of characteristics that determine the value and usefulness of data for its intended purpose.
Several vital elements can define data quality:
Accuracy: Data is considered accurate if it is free from errors and inconsistencies and reflects the actual state of the real-world phenomena it represents.
Completeness: Data is considered complete if it includes all the necessary information to support its intended use.
Consistency: Data is consistent if it adheres to defined standards and rules, such as formatting, data types, and naming conventions.
Timeliness: Data is considered timely if delivered promptly and reflects the current state of real-world phenomena.
Validity: Data is considered valid if it conforms to the business rules and constraints set by the organization.
Uniqueness: Data is considered unique if it can be distinguished from other data in the dataset.
Data quality is critical to data management, and organizations must ensure that their data is accurate, complete, and consistent. This can be done in several ways: data validation, data cleansing, data governance, data profiling, and monitoring.
Ensuring data quality is essential for organizations, as poor data quality can lead to inaccurate business decisions, wasted time and effort, and decreased customer satisfaction.
Data enrichment is essential for several reasons:
It can make data more valuable and useful: Adding additional information to existing data sets can provide a complete picture of the data and make it more beneficial for analysis and decision-making.
It can enable new insights and analysis: Enriching data with external data sources can provide new perspectives and insights that would not be possible with the original data set alone.
It can support compliance and regulatory requirements: Enriching data with additional information can help organizations comply with data privacy and security regulations, such as GDPR and HIPAA.
It can improve data quality: Data enrichment can improve the overall quality of data by adding context, correcting errors, and filling in missing data.
It can improve customer experience: By enriching data with customer information, organizations can provide more personalized and targeted services and enhance the customer experience.
It can support automation and decision-making: By enriching data with additional information, organizations can automate decision-making processes and improve efficiency.
In summary, data enrichment is essential because it can make data more valuable, enable new insights and analysis, support compliance and regulatory requirements, improve data quality, improve customer experience, and support automation and decision-making.
Data quality and enrichment aim to ensure that the data used for decision-making and analysis is accurate, complete, and relevant. This includes identifying and correcting errors or inconsistencies in the data and adding additional information that can enhance the value of the data. The goal is to improve the reliability and usefulness of the data and, thus, the quality of the decisions and insights that can be derived from it.